Predicting Game Difficulty and Engagement Using AI
Deep Reinforcement Learning technology set to be the new standard in game design and playtesting as well as predicting game difficulty and engagement.
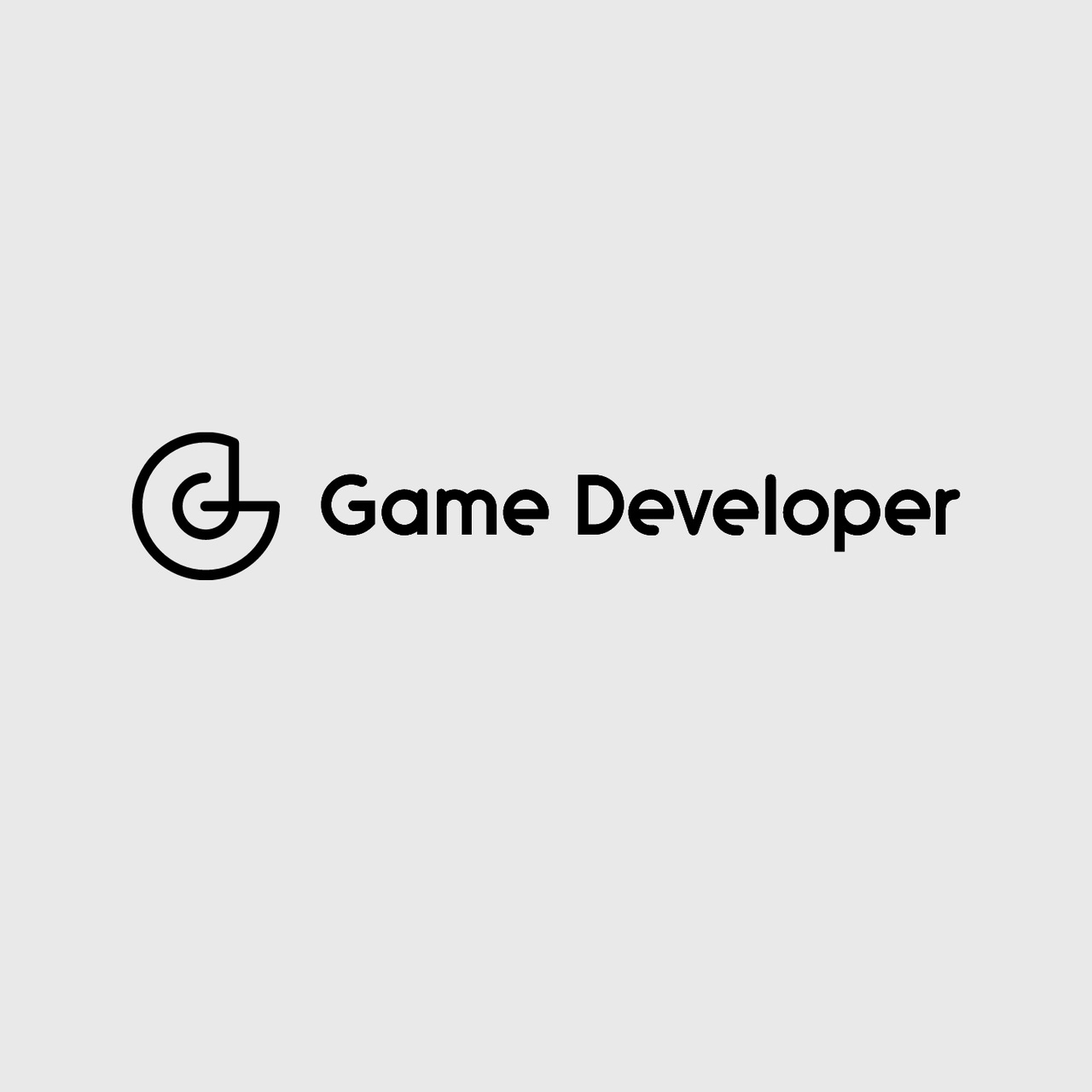
When talking about the future of gaming, it’s impossible to leave Deep Reinforcement Learning (DRL) out of the equation. Set to be the new standard in both development and innovation, DRL is also making waves in design and playtesting as well as predicting game difficulty and engagement. DRL takes on a whole new level as the industry is continuously undergoing radical transformation and market leaders are searching for new ways to streamline processes and cut noise where possible.
The main benefit of infusing DRL into these key processes over other traditional methods is that, instead of “manually” creating complicated behaviors or employing humans to perform exhaustive testing, DRL is quick to yield results by learning behavior and performing the necessary sequential steps to achieve the desired output.
Having an explosive growth in the demand for new games and content during pandemic, DRL has been introduced as a comprehensive method for automated gaming tasks, making it easier, faster, and more accurate for developers to work with clean, relevant data to improve games.
For example, DRL can be used to identify behaviors that would typically take place when a human was playing the game by means of virtual advanced players, who make intelligent associations between things or events happening in a specific environment. Different from other forms of learning, DRL goes beyond what’s traditionally known. It not only learns and determines how to make associations, it dives deeper into examining how to modify behaviors to achieve a desired output or avoid an undesired outcome. In a nutshell, it’s strategically growing smarter to optimize itself.
Game development for one, is greatly benefited by the ability to predict game difficulty and how players will engage with the game throughout levels as they evolve and progress their journey in the game. AI gameplay with Deep Reinforcement Learning can help simulate how players will pass levels and predict the level difficulty which can be used to emulate the required levels of skills, persistence, and when the player might leave the game out of lack of interest.
This data allows game developers to create comprehensive models of how players across different expertise levels will perform to specific levels of difficulty; also, this information helps understand which types of players are more likely to be less interested in the game or which will engage heavily with the playability of the game.
Player behavior predictions obtained from AI and DRL help shape gameplay and improve it through simple level simulations of player differences without the costly and resource-straining involvement of human agents collecting data for each type of player.
Thus, DRL helps solve one of the most prominent game design and development pitfalls which is predicting how a user will actually behave. This is on the coattails of the gaming industry that is consistently in need of better tools for prediction, user and player modeling, prototyping, and business optimization to determine why players sometimes don’t return to play the game.
For example, if you’d like to understand why a user quits after a specific level, DRL can generate predictive models of engagement and churn that allow game developers to conduct initial testing and prototyping via computer simulation.
Ultimately, DRL gameplay simulates how players might engage and operate with a game via computational models across different areas, mentioned above, like skills, persistence, and when the player might leave the game out of lack of interest.
All of these areas are improved by data-driven initiatives powered by AI, in specific, DRL.By using virtual, automated agents, game developers save money and time as tasks are performed in a fraction of the time as opposed to human agents. Of course, these automated agents need extensive training and learning exercises with large sets of player data to yield more accurate results. Without player data, DRL algorithms and models are somewhat blind and cannot generate realistic predictions of player behavior and experience.
The need for human playtesters had reached a point where the practice was becoming expensive, slow, and inaccurate. While very valuable yet with many limitations, human playtesters are becoming a thing of the past.
Now, AI-powered agents can get work done that was typically done in weeks, in a matter of hours, all with superior accuracy. These virtual, AI-powered agents are especially good at finding bugs, game parameter tuning, player pass rate, and difficulty prediction in game levels.
As expected, all players are different and all prefer different levels of difficulty. Virtual agents are capable of determining the level of difficulty and the effect said difficulty will have on player experience. This is extremely valuable for game developers as it helps improve the playability and performance of the game.
Through specific DRL algorithms that stem from machine learning, neural networks, and optimization principles, a virtual agent can observe the environment of a game and take action accordingly, which leads to further observation and a desired output. Through optimization, the agent finds the optimal path forward to maximize the amount of desired outputs or rewards.
When it comes to DRL in gaming, we believe this is the tip of the iceberg and that much more can be explored and leveraged to improve gaming experiences.
DRL enables game developers to explore and exploit the nuts and bolts of a game, increasing coverage and iterations, scaling with ease, and receiving feedback faster to improve the game as quickly as possible for end-user consumption.
Read more about:
BlogsAbout the Author(s)
You May Also Like