Using personalization to improve engagement and monetization in F2P games
A guide to using personalization to improve engagement and monetization in F2P games
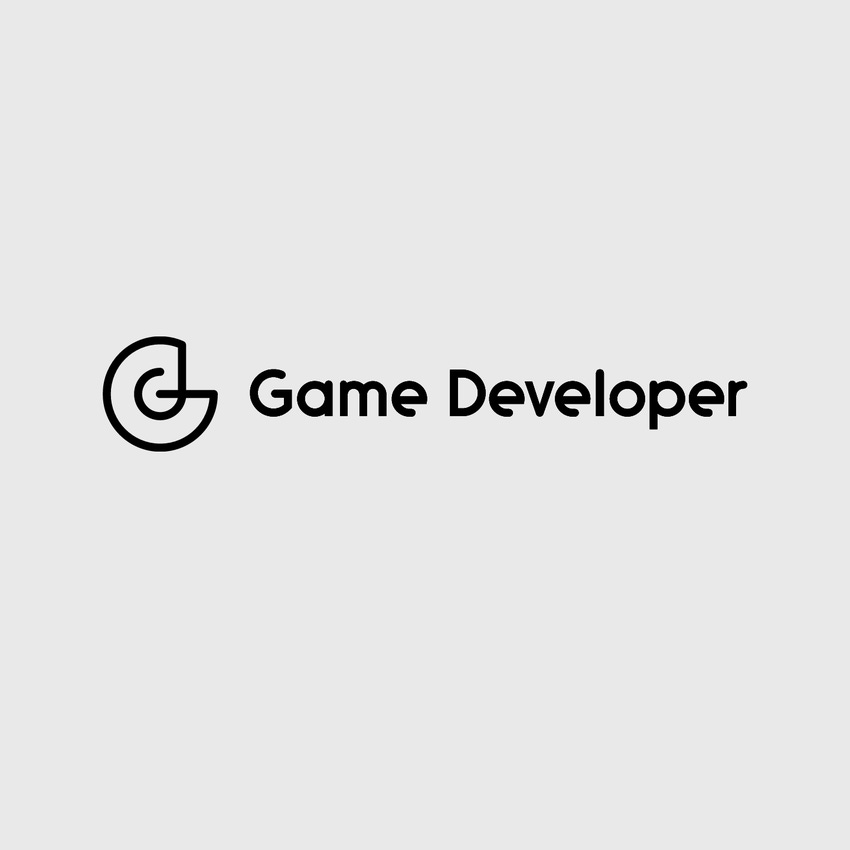
Treating players the same means that you end up providing a poor experience to almost all of them! Balancing retention and monetization is tough, however, our industry is rapidly improving it's use of data to solve these difficult challenges. Combining great experiences with rest payment and repeat spend requires insight, experimentation and focus.
The ‘Threshold of Engagement’
It would be very difficult to design an individual experience to engage each of your players. Instead, you need to use segmentation to and groups of players who share similar characteristics, and design game changes for that group. This process of personalization is underpinned by understanding that there is a threshold of engagement for all players, existing on a scale between boredom and anxiety. If players cross this threshold, it will cause them to leave the game.
Developers need to keep each player segment within the threshold by adapting the gameplay and marketing to suit their needs.
The first advantage of treating segments differently is that your players will enjoy your game more. A player whose experience is tailored to what they enjoy – whether that’s fast game play, building up coins, tricky puzzles, or plenty of hints – will keep playing and is more likely to monetize. While it’s not possible for every game to appeal to every audience, a fixed difficulty curve, for example, excludes a huge group of players who could love your game but are just a little too experienced or inexperienced for it.
And it’s not just player enjoyment that can be improved. Applying a real-time personalized approach to the monetization experience can dramatically increase profitability, by motivating players.
Predicting churn & personalization
So, we now know that players leave if they are anxious or bored, and we can see from the Threshold of Engagement chart that this state changes as they make progress through the game.
The challenge is to know which players are susceptible to leaving at which part of the game and why. It sounds like a tall order, but deep data analytics can provide the answer.
Using event data, and by identifying the precise metrics that contribute most to churn, it is possible to build a statistical model and apply within your analytics system to predict the probability of churn for each player, for each day they play - so, indicating who’s at greatest risk of churning and when.
Building the model
We would typically track a month’s worth of new installs through their engagement with the game, so the actual dataset for these players will be up to two months. Regression modelling is used to predict the influence a metric will have on churn, for each day’s play.
The number of metrics we test depends on the complexity of the game, but generically, the typical metrics that contribute most to churn include:
Activity – How much do they play?
Competency – Are they good at the game?
Spending – Do they have the right ideas for doing well?
Progression – How quickly do they progress?
Using our deltaCrunch demonstration game as an example, we use the model to calculate the coefficients that apply to each metric, for each day’s play, to identify the main influences within the game.
In this example, we can see that the level of activity is important throughout the early days, but its influence declines and becomes negative in days 4 and 5, telling us that we need to make the game easier at the start, but more challenging further on, as players may be leaving through boredom or lack of challenge.
Spending doesn’t seem to affect retention at the start, but from day 1 to day 3, it appears that players are being turned-off by the lack of value from grind currency expenditure, whereas the influence of this metric declines as the game progresses. Furthermore, we can see that the progression metric shows that we should slow down the gameplay for players who are progressing through the game too rapidly, as it makes a significant contribution to churn from day 4 onwards.
The specific metrics used, and supplementary data mining will provide much greater clarity and direction, helping with targeting specific mitigations to specific players.
Personalized testing of game mechanics
Once you’ve identified which metrics you need to improve, and which player groups you need to target, the next step is to come-up with potential remedies and test whether they work.
One advantage with churn prediction modelling is that it provides very specific objectives for evaluating your AB tests, you know who to target, which metrics you need to improve and where within the game you need to apply the changes.
It’s important to ensure you have enough data to be sure of the results. Often with games that have lower player volumes, or more highly de ned segments, patience is the key. For the same reason, don’t try to run too many tests at one time, as you’ll just have to wait longer to achieve the data volumes, and may slow down the process by missing-out on the potential to iteratively adjust each subsequent test based on what you’ve learnt.
When it comes to evaluation, we would always advise testing against a control group, so you can properly compare your results on other KPIs. For example, a gift may improve retention, but does it encourage or harm LTV? The potential to understand longer term implications of changes is another good reason to be patient with your testing.
After you’ve made changes to your game, you will need to re-calibrate your churn prediction model. The selection of metrics remains the same, but their coefficients will require adjustment.
Personalized monetization
We’ve looked at how to identify players who are susceptible to churning from their in-game metrics, and how to target those players with mitigations that are optimized through testing. While the principles of this approach can be applied to improving any game mechanic, such as rewards, offers and daily play mechanics, it is important to consider how you balance the use of your monetization tools, IAP, interstitial ads and rewarded ads so the in-game experience is maintained and the total in-game economy isn’t compromised.
The key to monetizing your whole game economy is prioritizing IAP. Bolting ads onto a game can be disastrous. F2P games that don’t optimize their ads so they synchronize with their IAP in a coordinated way can see significant cannibalization of revenues, some losing as much as 75% of ARPDAU.
Treating ads as a game mechanic and using them as part of your real-time player engagement toolset is essential. As with predicting churn, it is possible to predict whether a player is likely to make an IAP, and those that have the lowest probability should receive interstitial ads at the earliest opportunity, rather than using those slots for offers.
Grinders who decline IAP can be monetized through ad serving
At the other end of the scale, Whales, who are characterized by their high volume of purchases, should be encouraged to do so more often with IAP opportunities that support their style of play.
Fewer than 30% of players engage with rewarded ads, so there is a significant opportunity to make improvements, by testing their set-up, just like any other game mechanic. This may involve looking at elements such as the level of reward and how the mechanic integrates with the game, either to improve it for all players or to provide specific approaches, such as better signposting for only those players who aren’t engaging.
Knowing when to use which monetization mechanic, and who to use it with, can be the most important factor in monetizing using ads.
Read more about:
BlogsAbout the Author(s)
You May Also Like