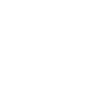
Featured Blog | This community-written post highlights the best of what the game industry has to offer. Read more like it on the Game Developer Blogs.
The Tenets of Community Management Series: Part 1 of 3
The Tenets of Community Management Series: Part 1 of 3
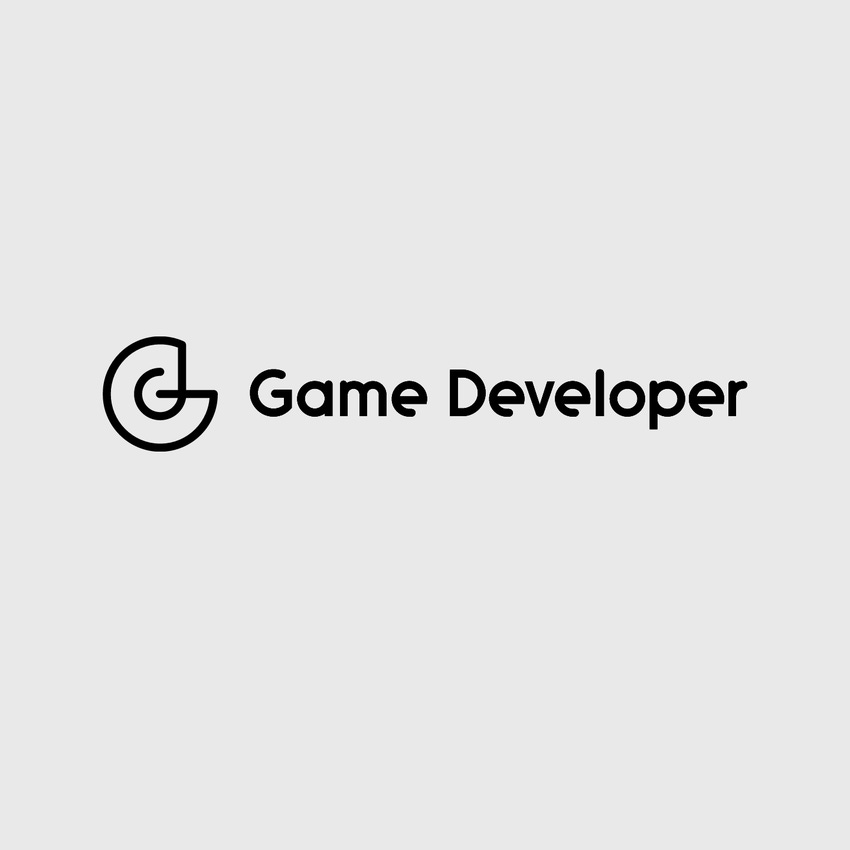
We’ve already established that user interaction is key to game monetization, and this series will take that concept one step further by discussing in-game community-driven monetization. This article will differentiate game community management from other forms of community management (social media, for example), and give an overview on how in-game communities can help or hurt developers. The introductory article will also prep users on how to build stronger gaming communities through a mix of tools, including predictive analytics.
Selling games to players is a great business. The only problem is that it’s not all of what you actually do. As respected developer Raph Koster has pointed out:
“It's a SERVICE. Not a game. It's a WORLD. Not a game. It's a COMMUNITY. Not a game. Anyone who says, 'it's just a game' is missing the point.”
That’s a big claim. Do the metrics bear it out?
Absolutely. Think about it this way: most games businesses operate and think as B2C, or business-to-consumer, models. A few are B2B. But what about C2C? Consumer to consumer, or in your case, player to player?
It turns out that players have a massive and important impact on each other—something community managers have always known, but have never been able to quantify. This Social Value™ actually accounts for usually 25-50% of all of your revenue. Social network analyses make capturing it possible.
Segmentation
It’s always smart to think about your users as being in different buckets and groups. You might guess that men will behave differently than women, that the French will spend differently than the Australians, or that the older users will send fewer messages than the younger ones. However you want to slice and dice it, segmentation lets you layer those group values on to other metrics.
Let’s stick with ARPPU for a minute. If the whole population is $25, but you find that men are $45 and women are $5, you’ve just diagnosed a problem. Segmenting can be done based on start date, which gives you cohort analysis. Or, you may segment based on one group you had see version 1 of the app and one group that saw version 2. Presto, you have AB testing.
More complex variations of segmentation allow multiple types. For example, show me the female Germans using iOS vs. the male Americans using Android. As always, the key is thinking about the right questions first, then picking the tool second.
Lowering Game Development Risks
As the video game landscape has evolved over the past few years from console-only to the explosion of mobile, the market is larger than ever. But a large and competitive market means an even larger financial risk for people who want to dive into the industry.
So how can a game developer minimize their risks? A new study by research firm SuperData surveyed over 40 video game companies to find out the best ways for lowering risks. The first conclusion in the study found that when determining a target customer base, a mix of B2B and B2C is the most effective: “Adopting a dual approach can play a role in derisking a business model by bringing in multiple revenue streams.”
Internally, the study found that it’s important to put an emphasis on partners to help game developers reach a larger market, while also valuing efficiency over creativity and innovation, to lessen risks and generate consistent revenue.
Increasing Player Retention with Predictive Analytics
In just a little over two years, the value of mobile game players has skyrocketed - in 2012, 1 million users were worth just $1.3M, and now they’re worth nearly $3.4M.
Since the value of mobile gamers is on the rise, it’s important to keep them coming back for more. Why? The market is becoming increasingly flooded, and more competition means that you’re at a higher risk of losing loyal players. It’s also more difficult and costly than ever to acquire new players.
One way to increase customer retention is by providing a solid customer relationship management (CRM) foundation - which includes customer support and overall community management. Couple that with a predictive analytics platform, and you’ll be able to analyze how it affects your bottom line, over time.
Take a look at this article by Julian Runge on GamaSutra, where he applies predictive analytics with a few customer retention tactics, to determine what has the biggest impact on players.
It's important to note that predictive game analytics are great, but you need a high confidence score to make the most out of any systems predictions. Not all platforms will show you this number. Depending on what platform you are using. It is worth taking a look at how accurate your predictions are. If you are just looking for an analytics platform now, it's important to ask these questions and determine if that platform will provide the accuracy needed to make real business decisions.
Gamer Differences
What drives gamer differences? According to a new study by research firm EEDAR, it’s platforms, not gender.
Thanks to the advancement of technology, the abundance of video game platforms has allowed players to experience more unique experiences than were possible 10 years ago. Now that we have mobile, PC, console and even VR, game developers are able to tailor their video games to a variety of ages and genders.
Predictive Analytics
Predictive analytics tell you the likelihood that something will happen. Again, you can think about this in the aggregate, or by individuals. Let’s say you want to know the likelihood of churn. You can see that value overall, or for each person. And, you should want to see their spending for context. The player who is 80% likely to leave and has spent $10 should not be treated like the player who is 70% likely to leave who has spent $2,000.
For example, what if you knew that player X is not going to spend any money for at least the next 10 days, you might think about taking some sort of action, right? If you knew that player Y is going to quit in 4 days and she's worth $100 to you, you’d do something to intervene. right?
Of course you would....if you only knew about it beforehand.
It's Like Predicting the Weather...But With People
As you delve into predictive analytics for games, you quickly discover that it's not radically different from forecasting the weather. You have data, you have trends, and you have an estimate for what’s going to happen tomorrow. The smartest meteorologists use scientific data-driven models. And over time you learn which ones you can trust.
As a game developer, you care about making predictions about people; specifically their likelihood to take certain actions. For example:
Churn
Where do people quit the game?
Why do they quit at that point in the game?
Why has churn rates increased (or decreased) recently?
Conversion
What makes players go from observer to freemium?
What makes players go from freemium to paying?
In-Game Monetization
What makes people decide to first start spending money?
What makes them spend more?
What makes them click on ads?
Player Lifetime Value
How much do players spend in total?
Social Value
What is the value of player's networks?
Are some players worth more than others?
Asimov's Predictions Are Your Reality
If you've read Isaac Asimov's Foundation Trilogy, you may remember that there's an advanced civilization that made a science out of understanding humans and societies so well that they could accurately forecast the actions of individuals.
But that’s science fiction. It's impossible to really predict the future, right?
Technically, no, it’s not impossible. And it's not magic either. It's math.
Luckily, the science here is getting easier to deal with as more and better tools become available. Do you need to hire a bunch of PhDs? You can but it’s not terribly practical (or cheap). Still, it’s important that you understand what actually happens so you understand how usable and actionable the results are.
Recognizing Patterns with Confidence
To start, let's keep it simple and imagine that only two patterns are possible in an adventure game.
Players login and play Level 1. Some go into the Dark Forest and 20% of them quit.
Players login and play Level 1. Some go into the Blue Bayou and 40% of them quit.
That’s pretty handy to know because it also starts to tell you that the Blue Bayou is in some sense, worse--and by worse, I mean it leads to quitting.
Now imagine a more realistic scenario where players are doing maybe 35 things and they do them in a wide variety of sequences. From this seemingly randomn series of events, sophisticated algorithms can mine the data and start to “learn” patterns.
This is why these programs are dubbed “machine learning.” Those programs then start to be able to look at a player and say, “based on what other players have done, there’s an 80% chance of this player converting or quitting or clicking an ad this week.”
And bam, you have predictive analytics!
In future posts, we’ll look at Individual metrics and cohort analysis as a further dive into this topic.
Read more about:
Featured BlogsAbout the Author(s)
You May Also Like