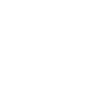
Featured Blog | This community-written post highlights the best of what the game industry has to offer. Read more like it on the Game Developer Blogs.
Improving user acquisition effectiveness with big data - Part 1 Predicting number of paying users by channel
User acquisition is very costly, so the earlier you know that a channel or source is not working the better. Learn how you can use big data predictive techniques to predict future payers from each acquisition channel.
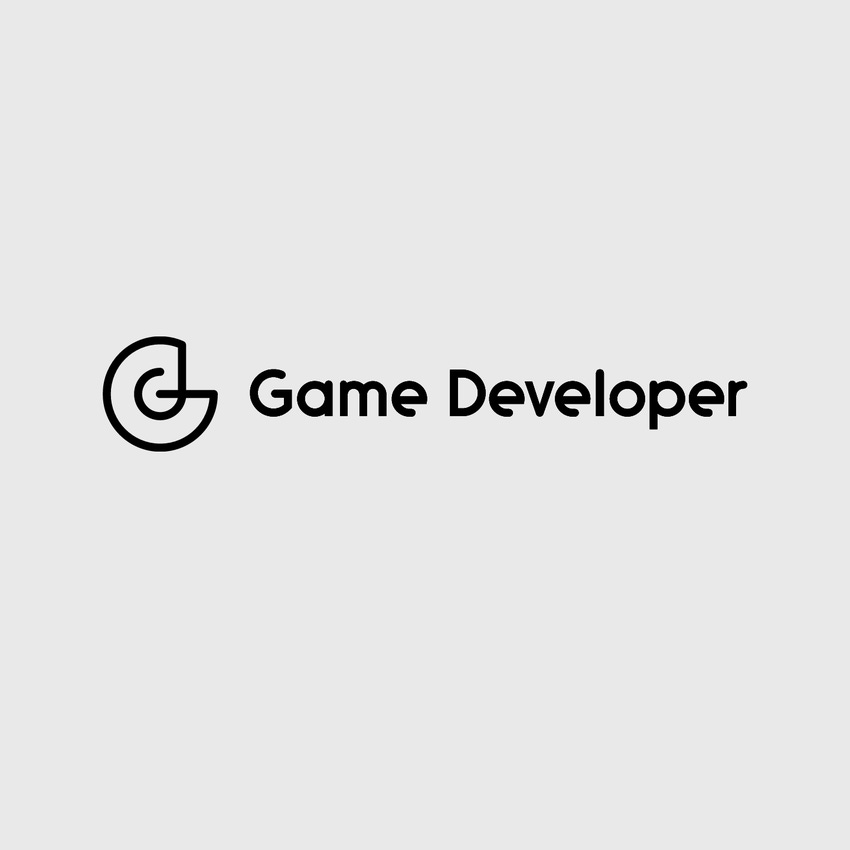
Introduction
Users are the lifeblood of games. To get a constant stream of players, developers and publishers are spending huge amounts of money, driving up the cost per user. The complexity is daunting as there are easily over 50 different places where you can spend money to acquire players. It is a never ending game, no pun intended, to find the channel that provides the lowest acquisition costs balanced with high quality players.
The question we will address in this article is how would we use big data to optimize the large amount of money that is used to fund player acquisition. We will assume that you are able to attribute players to the different acquisition channels, either completely or partially.
Framing the acquisition optimization problem
At the broadest level, one starts to compare the cost per player acquired and the value of each player. For example a recent article listed the cost per player for mobile games at $2.73 while the revenue per player was $1.96 In the quest to achieve a favorable balance, a first pass would be to look for channels with low acquisition costs. Let's start with an example where you spent $5000 to get 2000 users. The overall cost per user would be $2.50 Using attribution tools such as Mobile App Tracking or Google Analytics campaign ids, you can create a table that shows how the $5000 is broken down by the different acquisition channel. The table may look like this:
Channel | cost | # users | cost per user |
A | $1,000 | 500 | $2 |
B | $2,000 | 500 | $4 |
C | $2,000 | 1000 | $2 |
As you can see, channel B is more expensive from a cost per user perspective.
The next problem you will encounter is that not all users are equal; for a free-to-play game, a user who pays is vastly more valuable than a free user. In our example, let's assume that you got $3600 in revenue from those 2000 users, giving you an overall $1.80 revenue per user. So we can extend the table above to include the number of payers and the revenue from the payers.
Channel | cost | # users | cost per user | revenue | revenue per user |
A | $1,000 | 500 | $2 | 100 | $0.20 |
B | $2,000 | 500 | $4 | 2500 | $5.00 |
C | $2,000 | 1000 | $2 | 1000 | $1.00 |
Clearly even though channels A and C have the same cost per user, they are not bringing in the same type of paying users. And interestingly channel B which has the highest cost-per-user also has the highest revenue per user, thus justifying continuing to acquire users through that channel. This is of course somewhat exaggerated, but you get the idea.
One note about using average revenue per user: revenue per user actually follows a power law. Here's a previous post about this topic. So using average revenue per user can be tricky and unreliable. If you are concerned about that, you can instead calculate just the number of paying users.
Channel | cost | # users | cost per user | revenue | revenue per user | Current payers |
A | $1,000 | 500 | $2 | 100 | $0.20 | 3 |
B | $2,000 | 500 | $4 | 2500 | $5.00 | 20 |
C | $2,000 | 1000 | $2 | 1000 | $1.00 | 10 |
How about those free users?
Now the astute reader would have raised a couple of questions: (a) what about the free players? (b) what if the payers continue to spend in the future? (c) free users provide a good community of players in the game. Let us start with the free players...
Free players can provide a community and also might become payers in the future. The former is not easily quantifiable, but the latter is. We can use big data techniques to predict how many of the free users will become paying users in the near future. While we cannot go into depth on the technical aspects here, we can say that it is possible to accurately score free users on whether they will convert to paying status. (Interested readers can watch a 30min video on this at the Sonamine website) Based on these scores, one can generate a number of predicted future payers and expand the table further like this:
Channel | cost | # users | cost per user | revenue | revenue per user | Current payers | Future payers | Total payers | Rev per paying user | Cost Per Paying User |
A | $1,000 | 500 | $2 | 100 | $0.20 | 3 | 20 | 23 | $33.33 | $43.48 |
B | $2,000 | 500 | $4 | 2500 | $5.00 | 20 | 3 | 23 | $125.00 | $86.96 |
C | $2,000 | 1000 | $2 | 1000 | $1.00 | 10 | 25 | 35 | $100.00 | $57.14 |
The green column of "Future Payers" is the predicted number of future payers from each channel. Here are the other formulas:
Total payers = Current payers + Future Payers
Revenue per paying user = Total payers x revenue per user
Revenue per paying user = Total revenue / # payers (updated)
Cost per paying user = "Cost" / "Total Payers"
Because you have included the Future Payers in your calculations, the cost and revenue from each channel can now take into account the free users who might pay in the future. In our example, although Channels A and C have equal cost per user ($2), channel A would look to be a better choice because the cost per paying user is less than revenue per paying user that for Channel C (updated). Channel A seems to bring in users that convert to paying status at a later time. And so if you have another goal to boost the number of players without losing too much money, then channel A C would be better than channel C A.
Next steps
So what we have done is to estimate of how many paying users each channel will bring in, and by comparing the "cost per paying user" and "revenue per paying user", you can rank the quality of each acquisition channel.
So the next step would be to logically spend all your acquisition budget with the channel where the difference between cost per payer and revenue per payer is the lowest, right? No, every serious game marketer knows this won't work for several reasons : (a) the channel may not have enough inventory or users (b) buying more users from one channel measurably changes the cost per payer because it might drive up the bidding prices, and the additional users may not be of the same quality. Moreover, you may have different constraints to work with, such as looking for a minimum number of users to create a community.
So while channel B is very good for paying users, it's not bringing in a lot of users to begin with. If your game is "social" in nature, with PvP or sharing features, you must have a minimal number of users, free or paying at any time. So spending all your UA dollars on channel B is not an option.
In the next post, we'll address how to allocate an acquisition budget among all the different channels using the portfolio approach, take into account multiple different constraints and objectives, and have some fun with excel and linear solver...
Any questions, feel free to reach out [email protected]">[email protected] Thanks for reading!
--------
Update 2014 Mar 25
Thanks to Rick, who pointed out an error in the formulas and confusing comments.
Read more about:
Featured BlogsAbout the Author(s)
You May Also Like